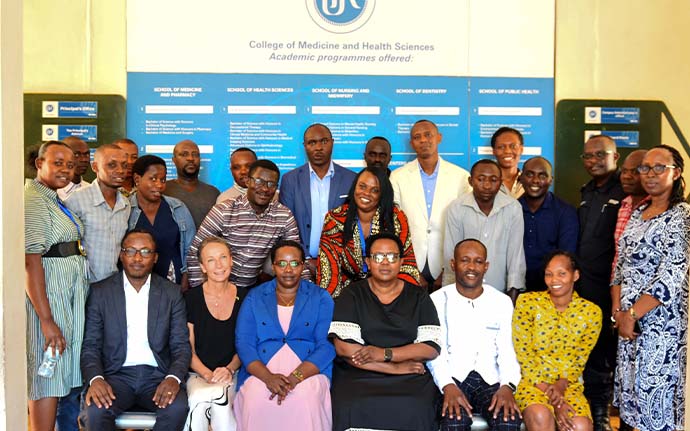
UR and Partners launch innovative research project to tackle urban mobility challenges in Africa
- 16-04-2025
- 1462
The World Health Organization reports that stunting affects approximately 37% of children under five in Sub-Saharan Africa, impacting around 58 million children. In Rwanda, childhood stunting has also emerged as a significant public health concern, but existing studies often fail to capture the nuances of regional variations. Global models lack the precision to address local stunting risk factors, limiting the effectiveness of current interventions.
This challenge led Gilbert Nduwayezu, a PhD candidate at Lund University under the Rwanda-Sweden research collaboration through the University of Rwanda, to conduct a study focused on childhood stunting in Rwanda’s Northern Province. His research, titled “Spatial Machine Learning for Exploring the Variability in Low Height-For-Age From Socioeconomic, Agroecological, and Climate Features in the Northern Province of Rwanda”, analyzed how socioeconomic, agroecological and climate factors contribute to regional variations in child height-for-age.
“We used localized machine learning techniques to identify the main factors influencing stunting prevalence,” Nduwayezu explained. Unlike conventional global models, which often overlook nuanced local dynamics, localized machine learning can uncover complex, non-linear relationships between stunting and multiple socioeconomic, agroecological, and climatic factors. This approach allows for a more understanding of the problem, revealing how specific variables interact to affect child growth outcomes in different communities.
Nduwayezu’s research revealed that 27% of children in the Northern Province of Rwanda are stunted, with the districts of Musanze, Gakenke, and Gicumbi showing the highest rates. These areas are particularly vulnerable due to a combination of different factors.
One of the most significant contributions of this study is its ability to provide insights that are directly relevant to policymakers. Nduwayezu’s use of localized machine learning showed that regional models were much more effective than global ones at identifying the complex interactions between height-for-age and influencing factors. These models highlighted specific thresholds of risk for stunting, allowing for the identification of the most vulnerable populations.
In conclusion, the study not only sheds light on the specific factors driving childhood stunting in Northern Rwanda but also offers a valuable tool for public health planning. By focusing on localized, data-driven insights, Nduwayezu’s research supports the broader efforts to reduce undernutrition and improve child health outcomes, contributing to Rwanda’s overall development goals.
Nduwayezu while presenting a poster of my research findings
Nduwayezu, a PhD candidate at Lund University under the Rwanda-Sweden research collaboration through the University of Rwanda